9. Lab: Pinhole Camera¶
9.1. What you will learn¶
- What the pinhole camera model is and how it is modelled with a projection in homogeneous coordinates.
- How to calibrate the camera, i.e. estimate the camera model parameters using the Direct Linear Transform.
9.2. Camera Calibration¶
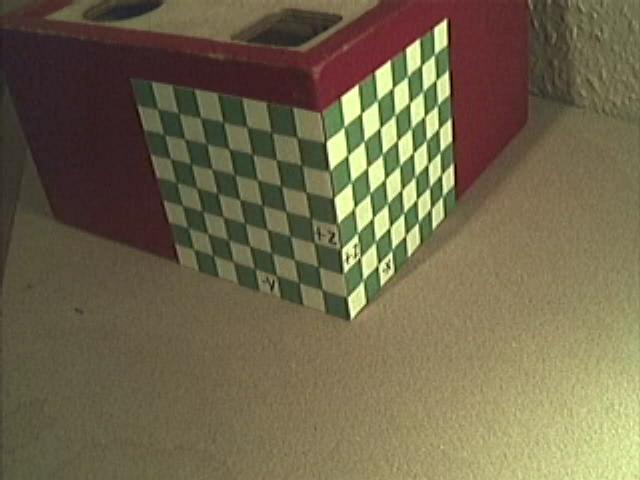
2D projection of a 3D object. On two sides of the box a checker board is drawn. The vertical axis is the positive \(Z\)-direction, the right side checkerboard is the plane \(Y=0\) and the left side checker board is the plane \(X=0\).
The goal of camera calibration is to find the numerical relation between the three dimensional points and the two dimensional points where these are projected. Thus we have to find the camera matrix \(P\) that projects points \((X,Y,Z)\) (in world coordinates) onto the 2D points \((x,y)\) in the image:
In this section it is demonstrated that, given the point correspondences \(\hv x_i \leftrightarrow \hv X_i\) for \(i=1,\ldots,n\), we have to solve:
Please note that \(\hv X_i = (X_i\; Y_i\; Z_i\; 1)\T\). The matrix \(A\) given above thus is a matrix in block matrix notation. For your implementation it is easy to first rewrite matrix \(A\) and use the components \(X_i,Y_i\) and \(Z_i\):
To find a null vector of \(A\) we can use the same ‘SVD trick’ that we have used to find the perspecive transform relating 2D points in one image with 2D points in another image (now we are relating 3D points with 2D points).
Given a null vector \(\v p\) we can reshape it into the required camera matrix \(P\).
In this exercise we will not discuss (and program) a way to
automatically extract points on the calibration object (the box with
checker boards that defines the 3D coordinate frame). These points are
already indicated (by hand). The matrix xy
gives the image coordinates
of several points on the left and right checkerboard. Every row in the
matrix is a 2D point. The corresponding points in 3D are given as the
rows in matrix \(XYZ\).
xy = array([[ 213.1027, 170.0499], [ 258.1908, 181.3219],
[ 306.41 , 193.8464], [ 351.498 , 183.8268],
[ 382.8092, 155.6468], [ 411.6155, 130.5978],
[ 223.7485, 218.2691], [ 267.5841, 230.7935],
[ 314.5509, 244.5705], [ 357.7603, 235.1771],
[ 387.819 , 205.1184], [ 415.3728, 178.1908],
[ 234.3943, 263.9834], [ 276.9775, 277.1341],
[ 323.318 , 291.5372], [ 363.3963, 282.1438],
[ 392.8288, 251.4589], [ 419.1301, 223.9051]])
and
XYZ = array([[0, -5, 5], [0, -3, 5], [0, -1, 5], [-1, 0, 5],
[-3, 0, 5], [-5, 0, 5], [0, -5, 3], [0, -3, 3],
[0, -1, 3], [-1, 0, 3], [-3, 0, 3], [-5, 0, 3],
[0, -5, 1], [0, -3, 1], [0, -1, 1], [-1, 0, 1],
[-3, 0, 1], [-5, 0, 1]])
In [1]: from pylab import *
In [2]: im = imread("images/calibrationpoints.jpg")
In [3]: imshow(im)
Out[3]: <matplotlib.image.AxesImage at 0x7f119bf65438>
In [4]: xy = array([[ 213.1027, 170.0499], [ 258.1908, 181.3219],
...: [ 306.41 , 193.8464], [ 351.498 , 183.8268],
...: [ 382.8092, 155.6468], [ 411.6155, 130.5978],
...: [ 223.7485, 218.2691], [ 267.5841, 230.7935],
...: [ 314.5509, 244.5705], [ 357.7603, 235.1771],
...: [ 387.819 , 205.1184], [ 415.3728, 178.1908],
...: [ 234.3943, 263.9834], [ 276.9775, 277.1341],
...: [ 323.318 , 291.5372], [ 363.3963, 282.1438],
...: [ 392.8288, 251.4589], [ 419.1301, 223.9051]])
...:
In [5]: plot(xy[:,0],xy[:,1],'d')
Out[5]: [<matplotlib.lines.Line2D at 0x7f119bf431d0>]
In [6]: axis('off')